A Note on Our Perspective of Data Automation
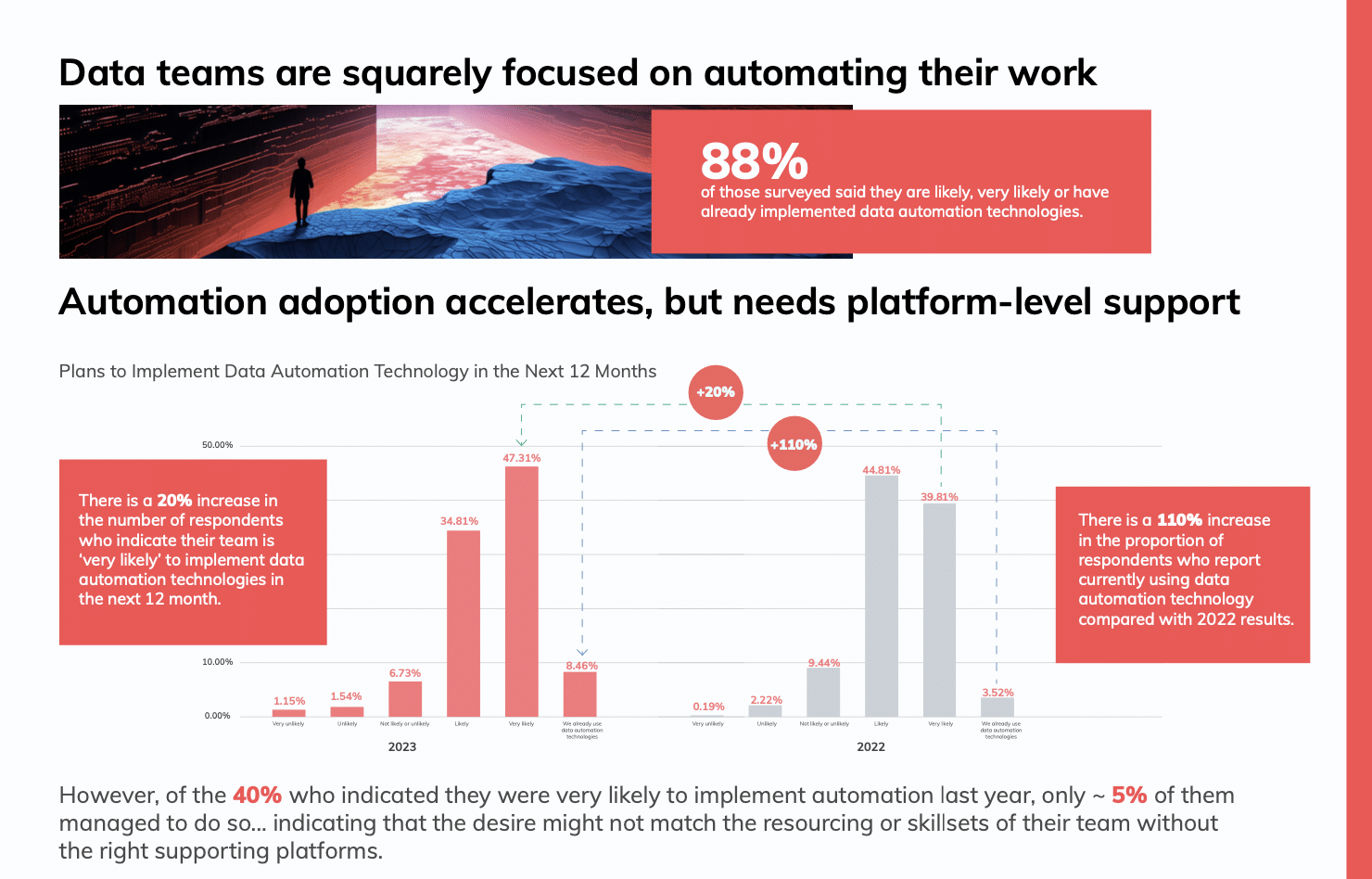
Ascend’s Fourth Annual DataAware Pulse Survey
Steps for Automating Data: A Detailed Walkthrough
Phase 1: Laying the Groundwork for Automation
1. Evaluate Your Data Ecosystem
- Consider Current Data: Begin by listing all your data sources. This could be spreadsheets, databases, CRMs, etc. Determine which data is critical for your operations and which might be redundant or obsolete.
- Determine Data Quality: For each data source, assess its accuracy, consistency, and relevance. Identify gaps, inconsistencies, or errors that might impede automation.
- Understand Data Flow: Once you understand where your data is coming from, define how it flows through your ecosystem. How is data currently being transferred from one point to another? Where is it stored? How easy is it to access business-ready data?
2. Establish Clear Objectives
- Define Goals: What do you aim to achieve with data automation? This could range from speeding up data entry processes, to ensuring data consistency, to near real-time data analysis.
- Expected Outcomes: Define the tangible benefits you expect from automation. Whether it’s a 20% reduction in data processing time or a 15% increase in data accuracy, having measurable outcomes can guide your journey.
- Stakeholder Involvement: Involve key stakeholders from different departments to understand their data needs and challenges. This collaborative approach ensures a more comprehensive automation plan.
3. Data Governance and Compliance
- Develop a Data Governance Strategy: This involves establishing who is responsible for what data and setting protocols for data access.
- Regulatory Compliance: Be aware of any data-related regulations in your industry. For instance, if you’re in healthcare, you’d need to consider HIPAA when automating patient data.
4. Integration and Workflow Design
- Map Out Desired Workflow: Before diving into tools, sketch out how you want data to flow once automated. Who inputs it? Where is it stored? Who accesses it and for what purpose?
- Address Integration Points: Identify where data needs to be shared between systems or departments. Think about how to make this transfer smooth, ensuring data integrity.
- Scalability: Consider the future. How will your data sources grow? Will the workflow you’re designing today accommodate tomorrow’s needs?
Read More: How to Ensure Data Integrity at Scale
Phase 2: Collaborative Implementation
5. Pilot Testing and Iteration
- Test on a Small Scale: Before fully automating, define the primary use cases you’d like to automate in a pilot or trial. This allows you to catch issues early and refine the process.
- Collect Feedback: During and after the pilot, gather feedback from users. What worked well? What challenges did they face? This feedback is invaluable in fine-tuning the process.
6. Prepare Your Team
- Educate Your Team: Automation often brings about change in how tasks are done. Ensure your team understands why changes are being made, and work with the platform to train them on any new processes.
- Provide Continuous Support: As the team adapts to automated data pipelines, be ready to offer support. This might involve troubleshooting, further training, or making tweaks to the automation workflow based on practical experience.
7. Monitoring and Maintenance
- Establish Key Metrics: Define what success looks like. This could be faster data processing times, fewer errors, or more timely reports.
- Schedule Regular Audits: Even post-automation, periodically review your data for quality and relevance. This ensures that your automated processes continue to add value over time.
Navigating Common Challenges When Automating Data
Embarking on the journey of data automation can be a transformative endeavor for many organizations. However, it’s essential to be aware of the challenges that may arise. By identifying and addressing these challenges proactively, you can ensure a smoother transition and a more successful implementation.
Data Quality and Consistency:
- Challenge: Even with sophisticated automation tools in place, the age-old adage “garbage in, garbage out” holds true. Inconsistent, outdated, or inaccurate data can compromise the results of your automation efforts.
- Solution: Regularly audit your data sources to ensure accuracy and consistency. Establish protocols for data validation and cleansing before integrating them into automated workflows. Look for tools that will automatically stop the propagation of bad data before it reaches end consumers.
Integration Complexities:
- Challenge: Data resides in various systems, databases, and applications. Integrating these disparate sources can become a significant hurdle.
- Solution: Make sure the platform you choose can act as a connector between different data sources.
Resistance to Change:
- Challenge: Resistance to change is a human tendency. Transitioning from manual processes to automated ones can face resistance from team members accustomed to traditional ways of working.
- Solution: Engage your team early in the automation journey. Provide training, communicate the benefits clearly, and ensure they understand the value automation brings to their daily tasks.
Defining Clear Responsibilities:
- Challenge: With automation handling tasks that were once manual, there can be ambiguity about team responsibilities.
- Solution: Redefine roles and responsibilities as necessary. Make it clear who oversees automated processes and who is responsible for intervening when things go awry.
Scalability Concerns:
- Challenge: What works for today’s data volumes and processes might not be suitable for future requirements, especially as businesses grow and evolve.
- Solution: Opt for scalable solutions and architectures. When designing workflows and choosing the platform you’re going to work with, always factor in potential increases in data volumes and complexities.
Real-World Examples: Automating Data in Action
Packaged Goods: Harry's
The Challenge
The Data Automation Solution
Impact & Results
"So we got out of the business of maintaining Python scripts, and got into the business of SQL transformations, which has been a huge upgrade for us in terms of maintenance and scalability."
William Knighting, Analytics Platform Lead at Harry's
Healthcare: Biome
The Challenge
The Data Automation Solution
Impact & Results
"We have been able to optimize our data pipelines, reducing processing time and enabling us to deliver data products faster. It has accelerated our cycle time and positioned us for future growth and scalability."
Stuart Jacobson, CEO at Biome